Tutorials
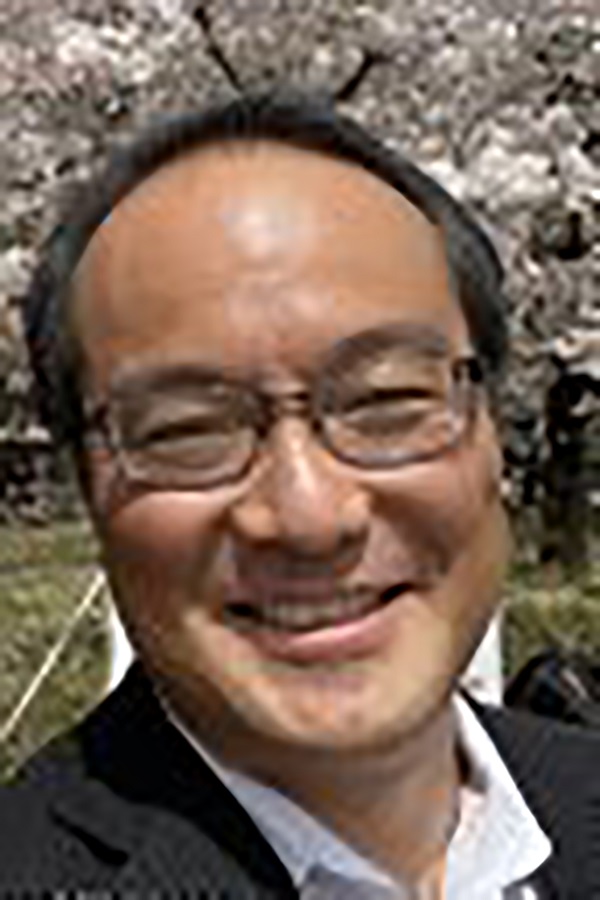
Takayuki Ito
Tutorial Title: Towards Hyperdemocracy: AI-enabled Crowd Consensus Making and Its Real-World Societal Experiments
Overview of tutorial: This tutorial will consist of following topics:
- Towards hyper-democracy: Augmented democracy platform by agent technologies (Takayuki Ito, Kyoto University)
- Developing Conversational Agents for Argumentative Discussions (Rafik Hadfi, Kyoto University)
- Argumentation theory for reasoning tasks (Ryuta Arisaka, Kyoto University)
- D-Agree: AI-enabled Crowd Discussion Support System and Its Real-World Societal Experiments (Sofia Sahab, Kyoto Unviersity) and (Jawad Haqbeen, Nagoya Institute of Technology)
Outcomes: In this tutorial, we are going to give talks about the literature of AI-enabled crowd discussion and agreement platforms and its real-word societal experiments. It starts from the vision and literatures on collective intelligence and crowd discussion support systems. Then, we go into the details on the development of conversational agents, which support crowd discussion. Finally, we are going to present the results on large-scale real-world societal experiments in Afghanistan. This tutorial is basically based on the insights we obtained in our two JST CREST projects.
Prerequisites for audience: The target audiences are students, younger professors, practitioners who are interested in AI, Multiagent systems, Collective Intelligence, Crowd Decision Making, NLP, CSCW, Large-scale Discussion, Consensus building.
Tutorial Details: https://sites.google.com/view/pricai2021tutorial
Presenter Bio: Takayuki Ito is from Department of Social Informatics, Kyoto University, Japan. He will act as the lead presenter of this tutorial.
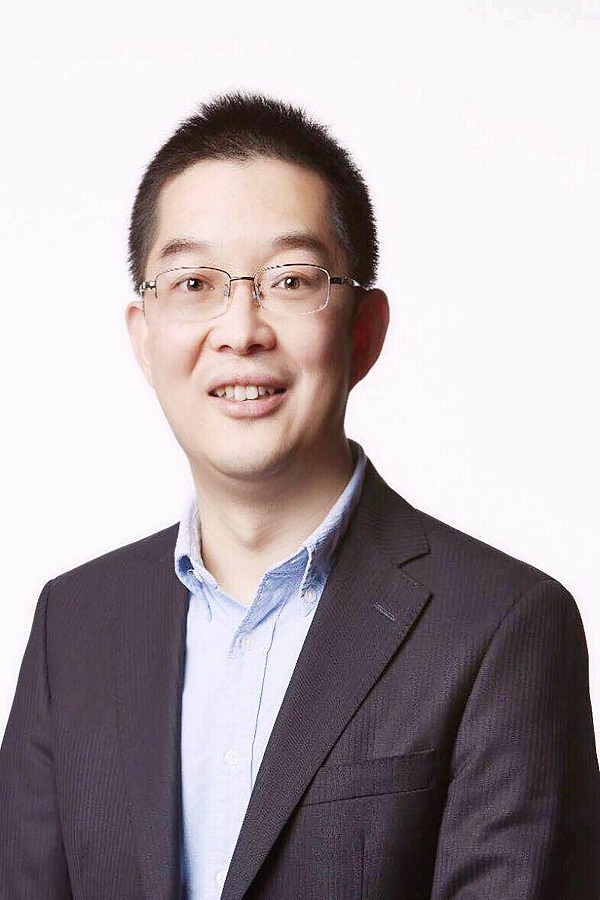
Kai Qin
Tutorial Title: Collaborative Learning and Optimization
Overview of tutorial: Computational Intelligence (CI) studies biologically and linguistically motivated computational paradigms and approaches to solve complex real-world problems that cannot be well handled by traditional methods. It is a hot sub-field of AI, consisting of three major pillars, i.e., Neural Networks, Evolutionary Computation and Fuzzy Systems.
Learning and optimization are two essential tasks that CI aims to address. Accordingly, many CI-based learning and optimization techniques have been proposed. In fact, CI-based learning and optimization are closely related. On the one hand, CI-based learning consists of various model-centric or data-centric optimization tasks. On the other hand, CI-based optimization is often formulated into learning-assisted search problems. In recent year, there emerges a new research frontier in CI, namely Collaborative Learning and Optimisation (COLO), which studies synergizing machine learning and optimisation techniques while unleashing unprecedented computing power (via supercomputers) to solve complex learning and optimization problems as well as real-world challenges in which learning and optimization are involved as interwoven tasks.
This tutorial will provide a comprehensive introduction to COLO from three aspects, i.e., how to make use of machine learning techniques to assist CI-based optimization (Learn4Opt), how to leverage optimization techniques to facilitate CI-based learning (Opt4Learn), and how to synergize machine learning and optimization techniques to deal with problems which involve learning and optimization as two indispensable and interwoven tasks (LearnOpt).
Outcomes: This tutorial aims to introduce to COLO, as a newly emerging research frontier in CI, which studies how to synergize machine learning and optimization while unleashing unprecedented computing power to solve complex learning and optimization problems as well as real-world challenges which involve learning and optimization as two interwoven tasks.
Prerequisites for audience: The audience is expected to have some basic knowledge on AI (particularly machine learning and optimization) and data analytics. Understanding CI techniques is not required but having an interest in knowing about CI techniques and using them to deal with real-world challenges that involve learning and/or optimization tasks may result in more take-aways.
Presenter Bio: Kai Qin is a Professor of Computer Science and Software Engineering, School of Science, Computing and Engineering Technologies, at the Swinburne University of Technology, Hawthorn, VIC 3122, Australia.
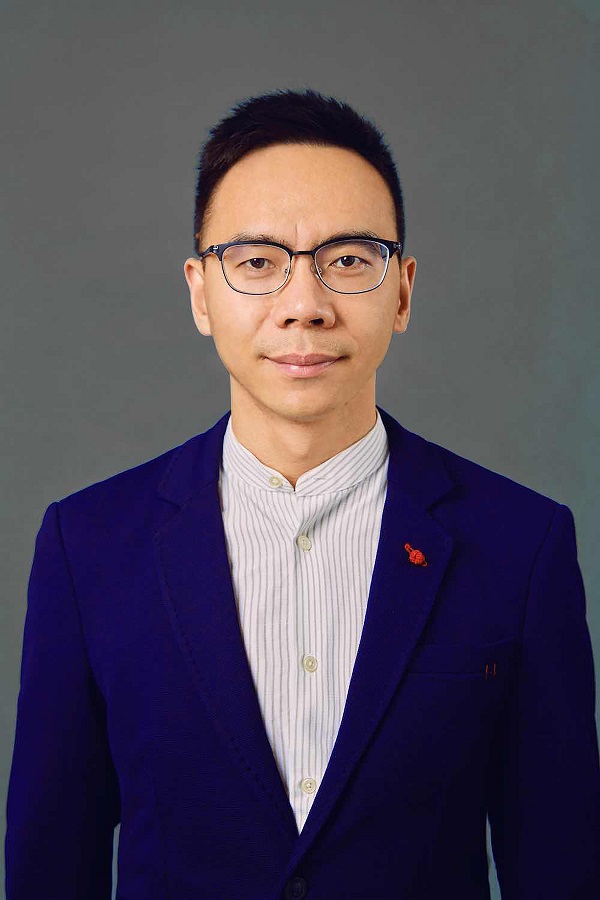
Dengji Zhao
Tutorial Title: Mechanism Design Powered by Social Interactions
Overview of tutorial: This tutorial introduces a novel mechanism design theory in social network settings to incentivize participants to invite their neighbours to join a mechanism/market. The challenge is that participants are competitors, and they would not invite each other by default. Traditional mechanisms such as VCG have not been designed for this purpose and new mechanisms are highly demanded in both theory and practice. Studies in recent years have shown that this is a promising research direction worth further investigation and this tutorial will overview the existing results and highlight open questions.
Outcomes: The literature of mechanism design has traditionally assumed that the set of participants are fixed and are known to the mechanism (i.e., the market owner) in advance. However, in practice, the market owner can only directly reach a small number of participants (her friends). To get more participants, the market owner often needs costly promotions, but the benefit received from the promotions may not even cover the costs of the promotions.
To solve this dilemma, one innovative way is to incentivize participants who are aware of the market to invite/inform their neighbors, and their neighbors would do the same. However, the participants are competitors, and they have no incentive to invite each other. Therefore, we need to design novel incentives to incentivize them to do so. The incentives can be achieved by giving them enough monetary reward, but that would give the market owner a huge deficit. Thus, the challenge is to design the incentives without sacrificing the market owner’s interests. This tutorial will discuss how to design such incentives in various settings especially resource allocation (auctions) and task allocation (crowdsourcing and coalitional games).
Prerequisites for audience: The potential target audience are PhD students and researchers who are interested in Algorithmic Game Theory, Mechanism Design/Auctions, Social Choice, Information Propagation on Social Networks. There is no strict requirement for attending the tutorial. Basic knowledge of game theory and mechanism design will be an advantage, but the basic definitions will be covered in this tutorial.
The audience will be familiar with the basic terminology of mechanism design and how it can be used in the new digital economy underpinned by social networks. Especially, the audience will understand the limitations of standard mechanisms such as VCG in the new settings and the new methods to overcome the limitations.
Presenter Bio: Dr Dengji Zhao is from Shanghai Tech University, Shanghai, China.